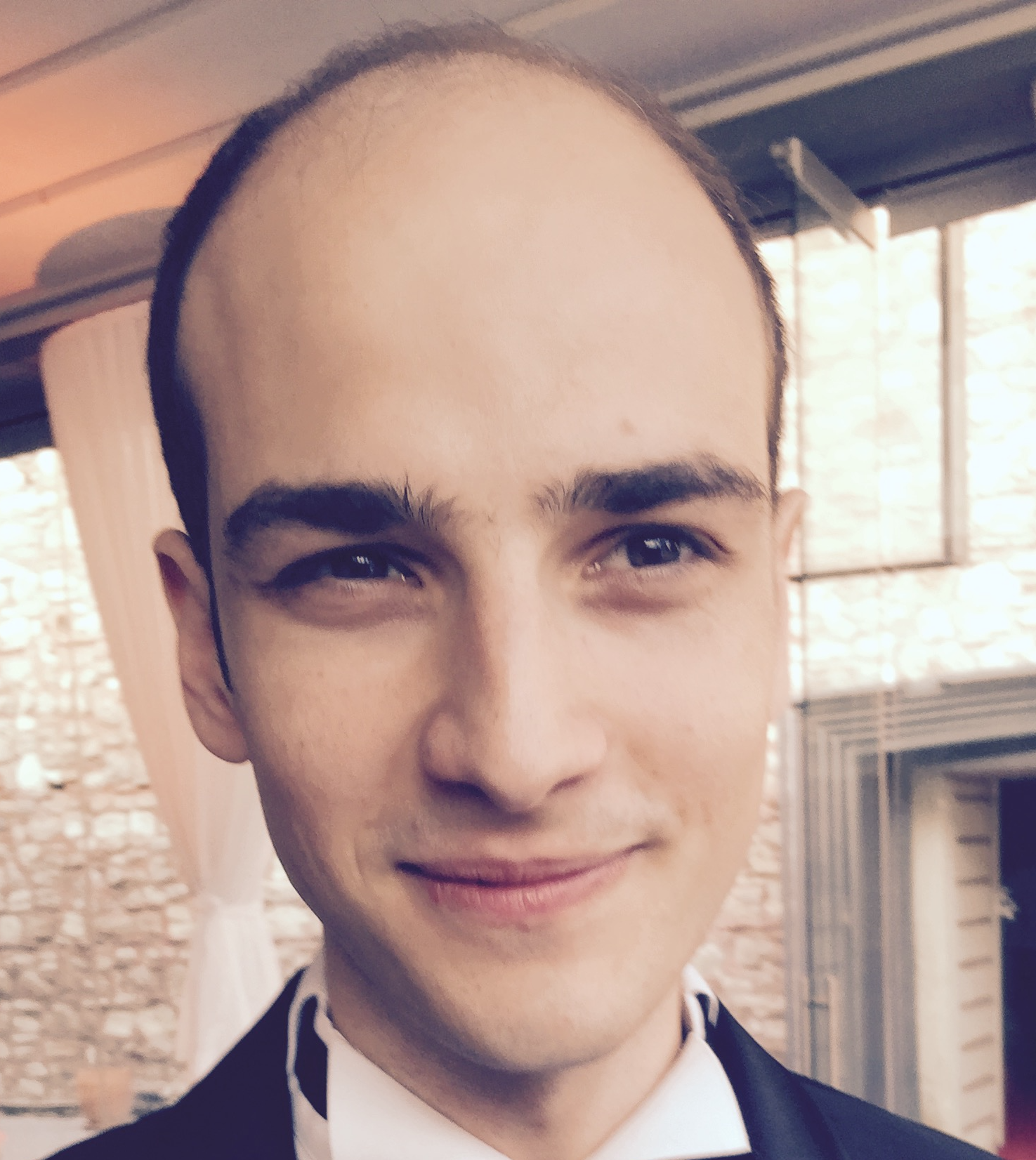
Rıza Alp Güler, PhD
I hold a PhD degree in applied mathematics and informatics from INRIA and École Centrale Paris. Previously, I have been a post-doctoral researcher at Imperial Collage London. I co-founded Ariel AI to bring my research ideas to products that will reach outside academia. We continue our journey in Snap, where I currently work as a computer vision resarch engineer and manager. We enable the next generation of augmented reality experiences with 3D human bodies.
An up-to-date list of my publications can be found in Google Scholar.
-
Our paper on bone-level parametric human body modellingn accepted at ECCV 2020 as a spotlight. Congrats Haoyang !
-
Our paper on in-the-wild hand reconstruction accepted at CVPR 2020 as an oral presentation. Congrats Dominik !
-
Two papers accepted at CVPR 2019 as oral presentations.
-
I receive STIC Doctoral School Best Sceintific Contribution Award 2018.
“DensePose” has received the second place (ex aequo) in the annual award for PhD student research projects. ( link ) The doctoral school includes many renowned French universities and “grandes écoles”, including CentraleSupélec, Ecole Polytechnique, ENS Cachan, Télécom ParisTech, Université Paris-Sud, etc.